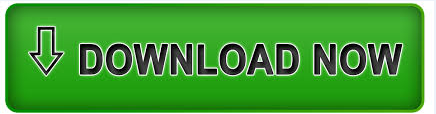
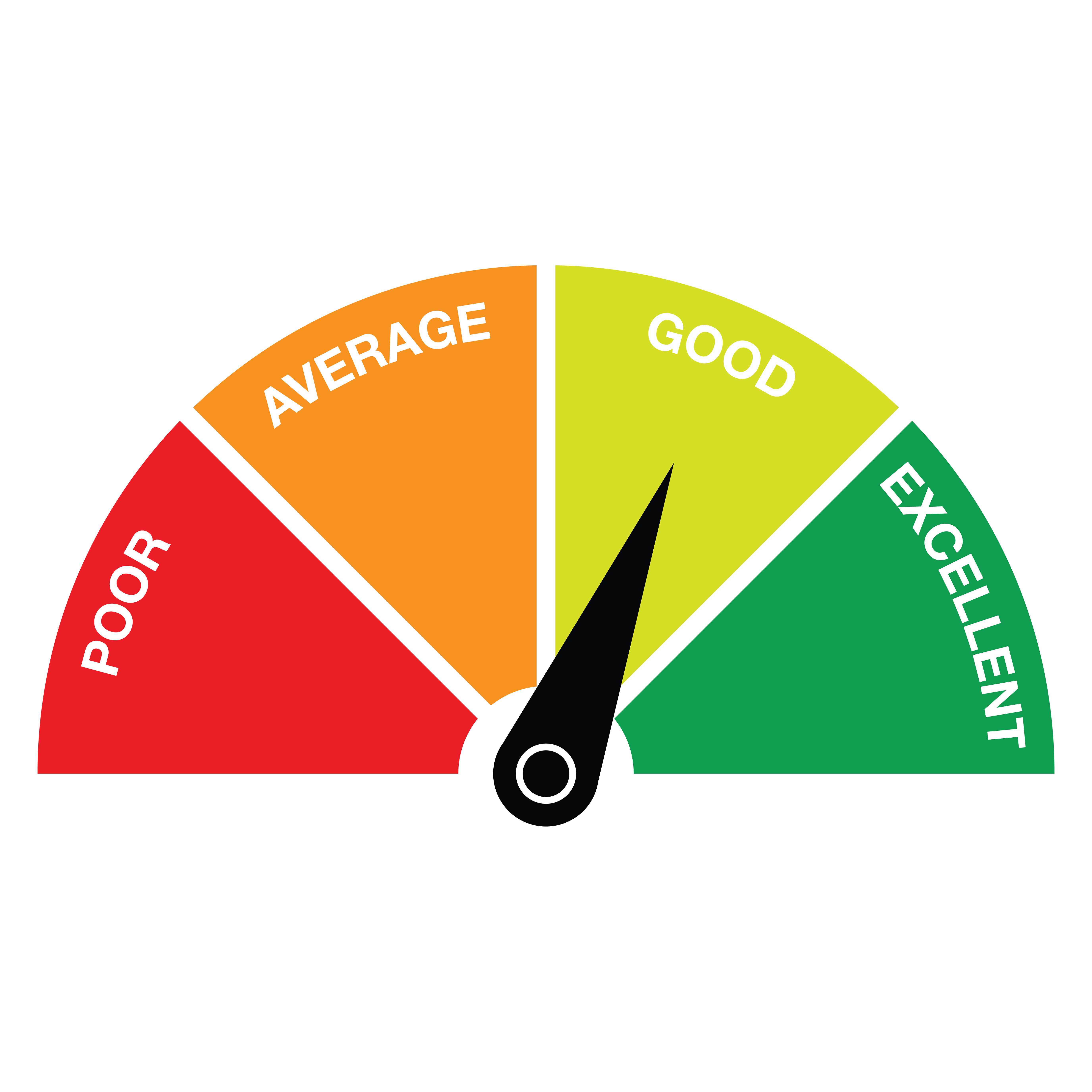

The rich body of sleep research using wearable devices has contributed substantially to our understanding on the importance of sleep to overall health 1 with poor sleep linked to the progression of many diseases, including depression 2, hypertension 3, obesity 4 and neurodegenerative diseases 5.
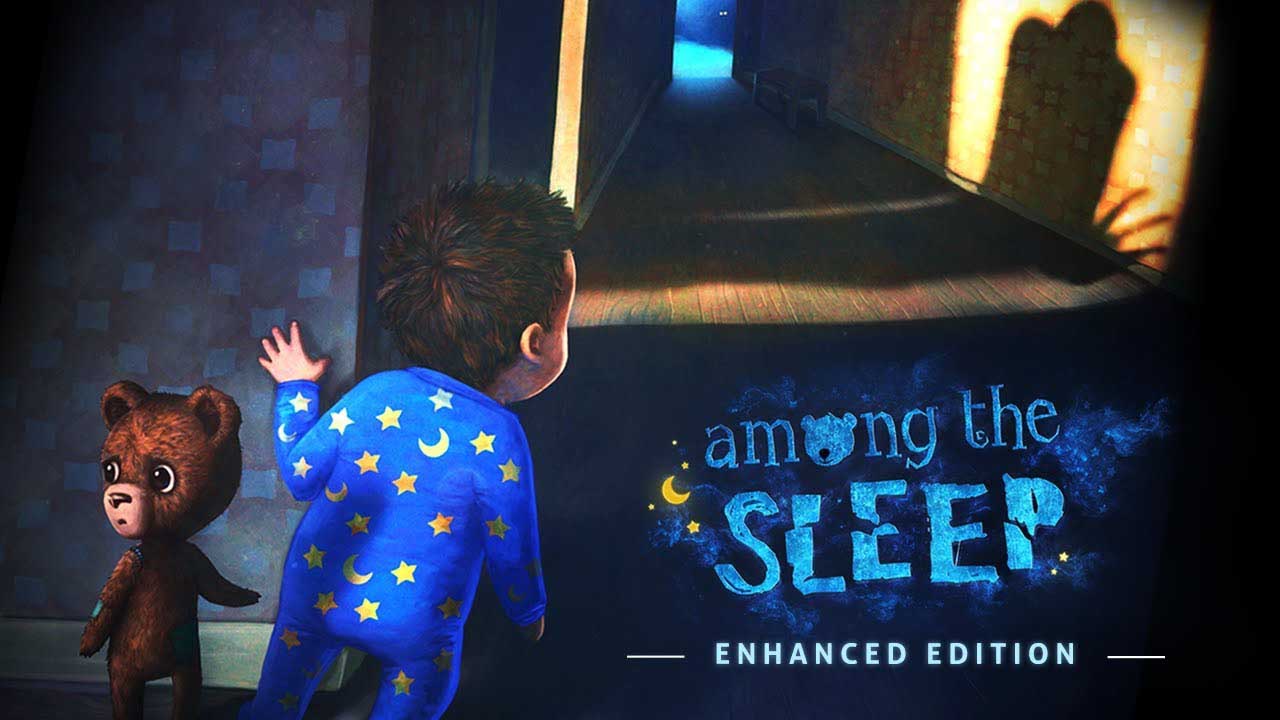
This research grew rapidly in the 90s with improved sensor technology, increased storage size and improved usability of the devices. With the advancement of sensor technology in the 1970s, sleep researchers started to experiment with the use of accelerometers worn on the wrist to objectively assess sleep and circadian patterns. Sleep has intrigued researchers in both basic and clinical sciences for more than a century. This independent and systematic analytical validation of sleep algorithms provides key evidence on the use of wearable digital health technologies for sleep research and care. The performance of complex machine learning and deep learning models seem to suffer from poor generalization. Despite the inclusion of recently published complex algorithms, simple regression-based and heuristic algorithms demonstrated slightly superior performance in sleep-wake classification and sleep outcome estimation. This work provides a systematic evaluation across 8 state-of-the-art sleep algorithms on a common sleep data set with polysomnography (PSG) as ground truth. However, it remains unclear how these algorithms compare to each other on the same data set and if these modern data science approaches improve the analytical validity of sleep outcomes based on wrist-worn acceleration data. Since the publication of the first automated scoring algorithm by Webster in 1982, a variety of sleep algorithms have been developed and contributed to sleep research, including many recent ones that leverage machine learning and / or deep learning approaches. The proper use of wearable technology in sleep research requires validated algorithms that can derive sleep outcomes from the sensor data. For the last 40 years, actigraphy or wearable accelerometry has provided an objective, low-burden and ecologically valid approach to assess real-world sleep and circadian patterns, contributing valuable data to epidemiological and clinical insights on sleep and sleep disorders.
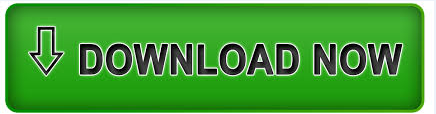